Federated learning constitutes an emerging privacy-preserving machine learning methodology that fundamentally enables organizations to collaboratively train AI models without necessitating sensitive data sharing across central servers. This decentralized approach warrants tremendous potential across diverse global sectors as intensifying data privacy regulations and localization necessities inhibit centralized modeling pathways dependent on open data pooling.
Across this discussion, I comprehensively outline the top 10 industries primed to actively implement federated learning schemes over the next five years as a critical enabler to optimize models while retaining proprietary data custody and adhering to intensifying privacy policies. As decentralized networks and edge devices continue proliferating amid the global data revolution, federated learning provides a momentous pathway to collaboratively enhance models while allowing each node to maintain exclusive data control.
This data localization attribute resonates across numerous verticals and uses cases dealing with large, multifaceted datasets subject to confidentiality constraints. The decentralized federated learning paradigm shift thereby unlocks groundbreaking opportunities for cross-institutional and cross-sector modeling partnerships previously deterred by competitiveness barriers and data protection necessities.
1. Healthcare
Healthcare organizations worldwide recognize rapidly escalating regulatory, ethical, and policy necessities around patient data privacy and confidentiality. Meanwhile, medical datasets continue expanding exponentially. Federated learning importantly empowers privacy-preserving global research collaborations between hospitals, health systems, and regional networks to significantly enhance clinical diagnosis and treatment models without necessitating any distribution or compromise of locally controlled sensitive patient information. Multi-institution partnerships can jointly advance imaging analytics, genomic sequencing models, patient monitoring systems, and population health indicators using federated learning while allowing each institution to retain exclusive localized data custody at all times by localized policies.
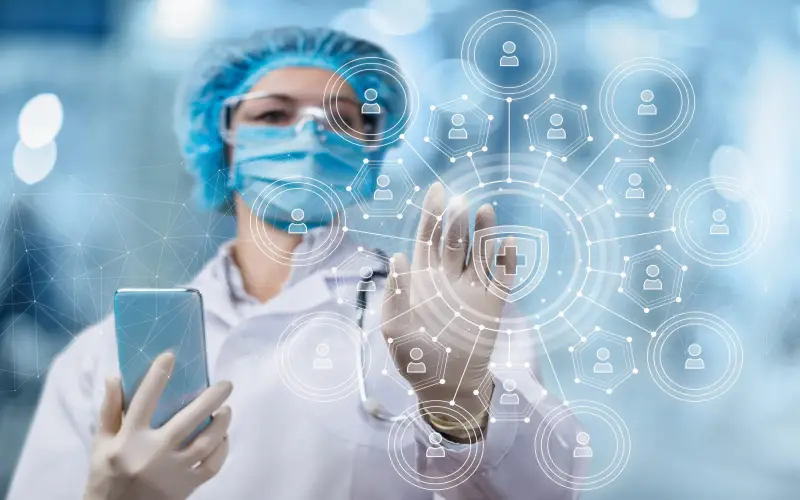
2. Telecommunications
Individual telecommunication providers including mobile operators and internet service providers capture and manage immense datasets around consumer usage patterns, infrastructure performance indicators, network traffic analytics, and customer activity records. While significant potential exists for predictive modeling, competitive realities severely stifle incentives for firms to merge datasets into open pools. However federated learning importantly unlocks extraordinary new opportunities for telecoms to cooperatively enhance shared models for optimized 5G infrastructure, fraud detection, churn propensity identification, and customer experience optimization without necessitating any raw data consolidation outside enterprise firewalls.
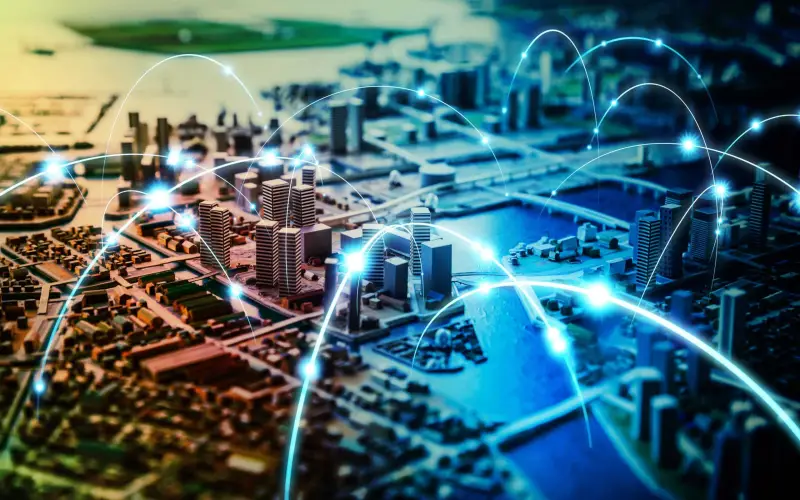
3. Transport
Mobility datasets across transportation sectors amass rapidly with the proliferation of sensors and telemetry systems across connected vehicles, city infrastructure, consumer devices, and logistics endpoints. However, these datasets remain tightly protected given the commercial sensitivities of rider statistics, traffic patterns, autonomous vehicle indicators, and freight metrics. Federated learning constitutes a breakthrough solution allowing both private and public sector mobility stakeholders including rideshare platforms, vehicle manufacturers, smart city developers, and transport agencies to cooperatively refine predictive models for traffic optimization, efficient routing, infrastructure planning, fleet electrification coordination, and predictive maintenance without necessitating any actual merging or centralization of highly sensitive raw datasets.
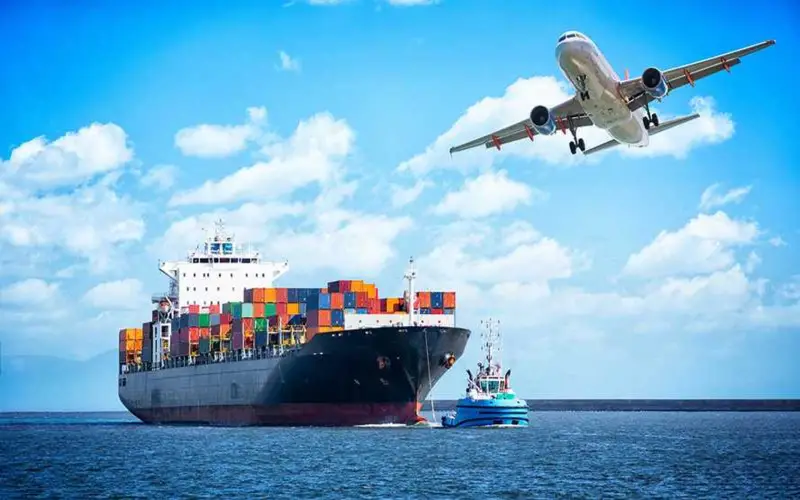
4. Manufacturing
Industrial IoT platforms across automotive plants, aerospace factories, appliance assembly lines, and other manufacturing facilities generate immense datasets ripe for optimization – but collectivizing real-time production statistics with outside vendors or partners faces barriers. As competitiveness considerations strictly prohibit the pooling of confidential operational data outside corporate firewalls, federated learning critically enables decentralized optimization across manufacturing networks – allowing multiple institutions to jointly enhance automation systems, digital twin models, supply chain coordination algorithms, and predictive maintenance tools federated without moving any proprietary data whatsoever beyond on-premise enterprise custody at any point.
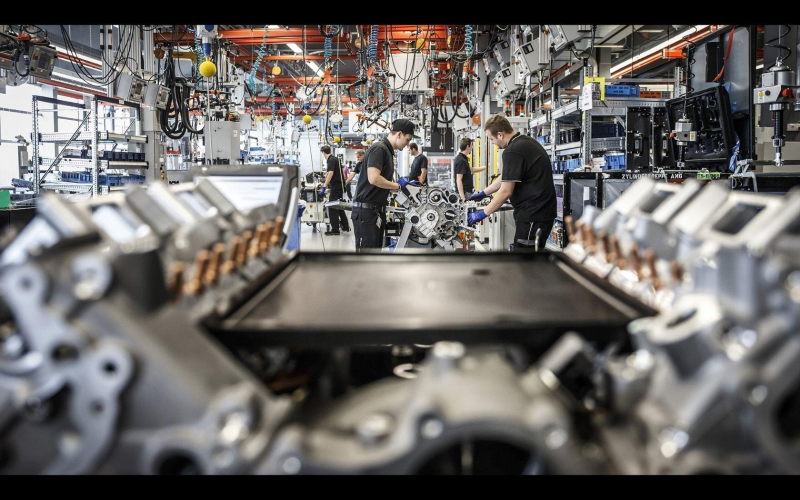
5. Retail
With immense digital adoption and intense competition, retailers across segments capture billions of customer transaction records daily representing highly sensitive datasets once aggregated. While significant shopper behavior insights reside siloed across grocery chains, general merchants, specialty retailers, and local stores, pooling data is never possible. However federated learning importantly facilitates both intra-enterprise and collaborative modeling opportunities for retailers to collectively uncover shopper insights, inventory demands, and personalized recommendations without necessitating co-mingled transactional records or violating customer confidentiality.
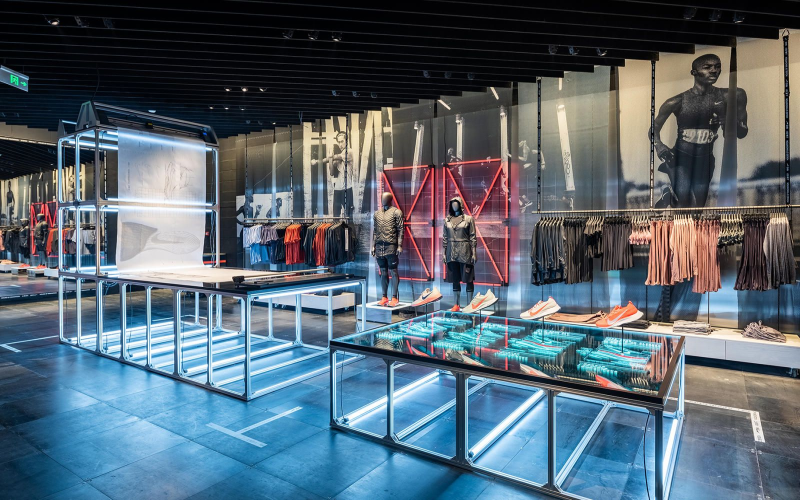
6. Finance
Global banks, insurance providers, and financial service institutions securely manage immense consumer data assets increasingly subject to more stringent regulatory controls amid a complex cross-section of geographic jurisdictions and sectoral policies. With fraud and cyber threats compounding daily alongside market volatility risks across lending, insurance, and trading – federated learning aptly constitutes a monumental privacy-preserving pathway for decentralized risk analysis, portfolio optimization, fraud detection, regulatory model co-development, and even sentiment analytics across financial sectors without violating fundamental data custody and confidentiality duties.
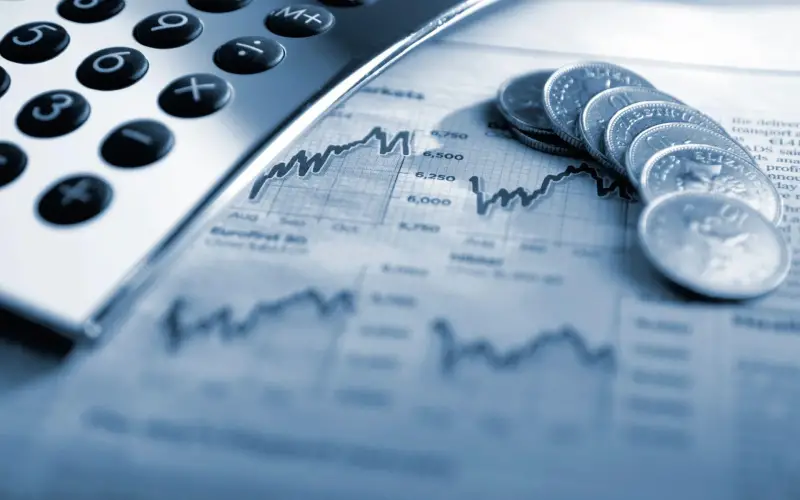
7. Education
Academic researchers, higher education institutions, and credentialing providers across all pedagogical domains recognize escalating necessities – and regional requirements – around ethics, transparency, and privacy policies restricting any distribution of sensitive student performance records or research data outside secured servers. However enormous opportunities exist for collective modeling, assessment enhancement, and scalable learning optimizations leveraging datasets across multiple institutions, laboratories, libraries, and regional school systems. Federated learning importantly provides a monumental privacy-preserving avenue for education partners to jointly enhance learner analytics, student assessment techniques, instructor effectiveness metrics, high-stakes exam item calibrations, digital learning recommendations, and even collaborative multi-site research data harmonization schemes without necessitating any raw statistical distribution outside localized servers.
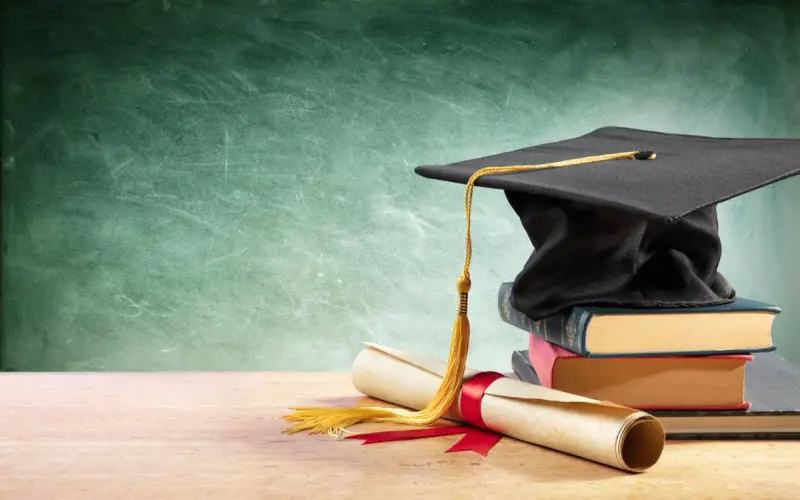
8. Entertainment
As digital streaming platforms continue expanding user bases while gathering immense viewer engagement statistics – and creative enterprises from film production houses to music labels retain closed custody of highly confidential pre-release scripts, footage, segment tests, and plot trajectories – federated learning crucially enables cross-sector collaborative analytics around audience sentiment predictions, real-time viewership pattern recognition, content quality scoring, and creative optimization guidance without necessitating any co-mingling of raw viewership data or intellectual property assets across entertainment partners.
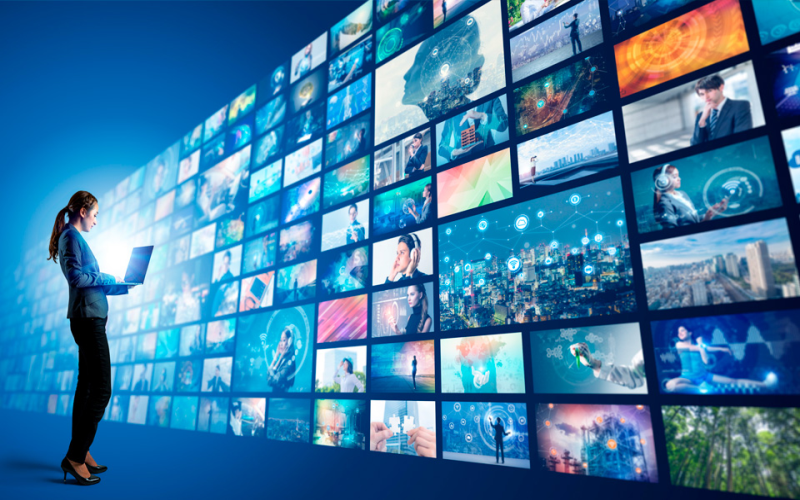
9. Agriculture
Localized on-farm yields, hyperlocal weather datasets, seasonal soil condition indicators, commodity pricing records, and farm vehicle sensor statistics remain tightly consolidated data silos concentrated across individual agricultural operations that realistically cannot be pooled into open data lakes without presenting competitiveness concerns or legal barriers around proprietary custody. However enormous potential exists for collective modeling – especially on precise yield prediction, early crop disease detection, livestock health pattern recognition, farm input optimization, and precision agriculture management systems – leveraging harmonized learning across regional agricultural networks.
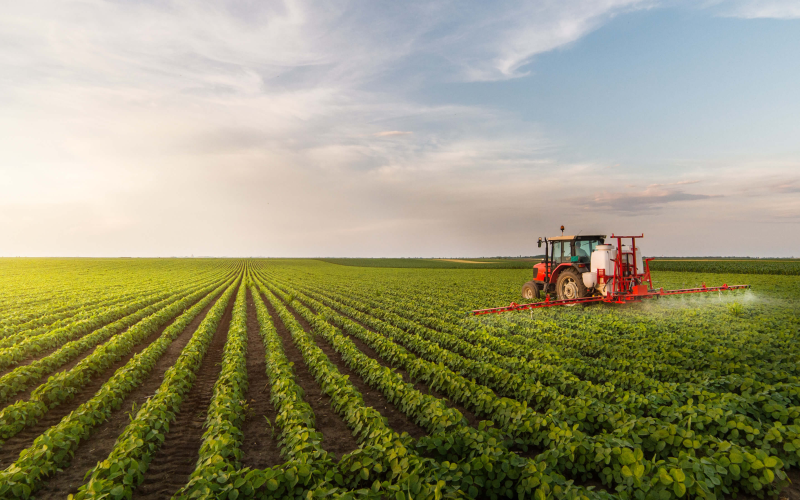
10. Energy
As utility providers operate amid highly sensitive privacy protections strictly prohibiting the sharing of individual household energy consumption data while power generators and grid operators retain confidential information around demand forecasts, supply bids, and infrastructure performance – immense opportunities exist for efficiency enhancement across the interconnected ecosystem. However, confidentiality barriers have severely constrained previous attempts at pooled modeling.
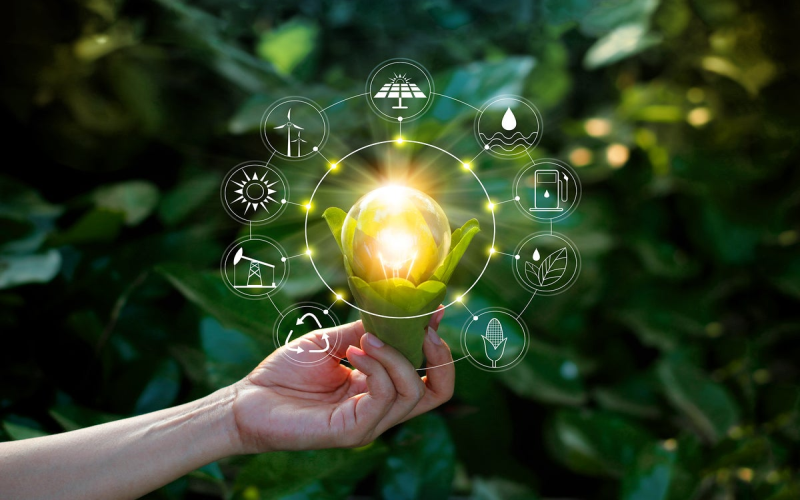